Hierarchy of Effects
Hierarchy of Effects systems capture customer engagement flows in an idealised sequence of stages, in order to plan marketing campaigns, design product funnels and develop metrics to monitor user progress towards product goals. Some notable examples are AIDA, REAN and AARRR.
As the hierarchies of effects essentially try to model similar parts of the user progress towards product goals, many of them overlap.
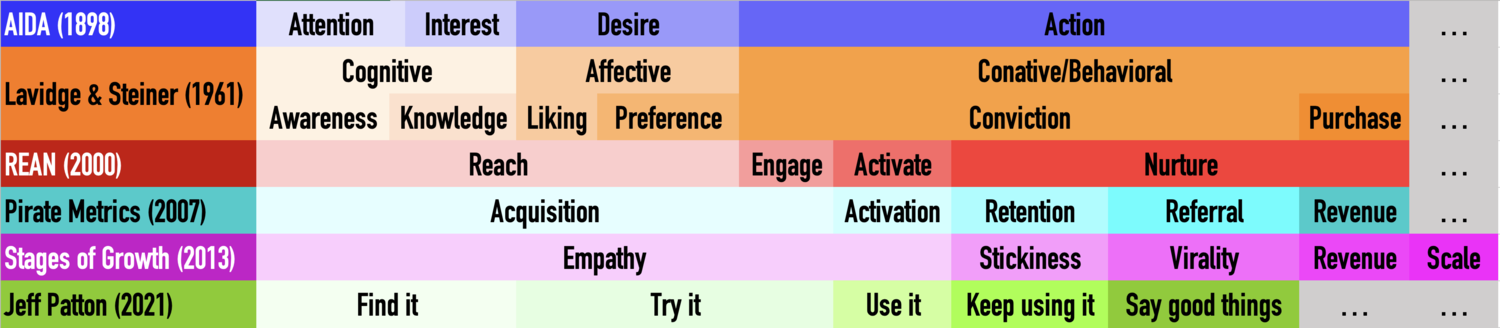
(open the image in a new tab)
Popular Hierarchy of Effects systems are similar in several ways:
- They are highly contextual, based on intuition and experience instead of scientific research.
- The names are usually a mnemonic based on the initial letters of each of the steps.
- They focus on a specific part of a user journey, not attempting to create a complete picture.
- They tend to describe the flow for a majority of users, and do not describe well situations when users skip certain steps, take them out of order, or take certain steps repeatedly in a cycle.
- They tend to inspire variants that reorder or add steps to expand the applicability to related contexts.
In general, such models have proven useful for discussions, planning and product decision making, as long as they are not taken too literally. Hierarchy of Effects systems are idealised abstractions, and they do not capture the real world accurately. Trying to use them too literally can be highly misleading.
Hierarchy of Effects systems are context-specific, not universal
Academic researchers in the areas of psychology and advertising have tried to create universal models for a hierarchy of effects for a long time, without success. Such attempts either end up creating highly abstracted versions that are no longer useful for detailed planning (such as Cognition, Affect, and Experience), or models that are so complicated that they can’t be applied easily, such as AISDALSLove.
Robert J. Lavidge and Gary A. Steiner in 1961 proposed a universal hierarchy of effects, based on the related to a classic psychological model dividing mental states into three groups, thinking, feeling and desires (cognitive, affective, and conative states).
- cognitive stage for mental or rational functions (Awareness, Knowledge),
- affective stage for emotional functions (Liking, Preference),
- conative stage for behavioral, striving and motivational functions, treating objects as positive or negative goals (Conviction and Purchase)
However, even this sequence seems to be context specific. Valenti and colleagues in the paper Advertising’s sequence of effects on consumer mindset and sales: A comparison across brands and product categories claim that the sequence varies across brands, and that the Affect - Cognition - Experience (ACE) sequence is actually the most common.
Hierarchy of Effect systems spawn variants
Because the models are so context-specific, it’s very common for popular models to spawn variants that remove, add or reorder steps. The new models tend to be presented as more complete or more universal, but in effect they just apply better to a different context.
In the The Development of the Hierarchy of Effects: An Historical Perspective article in 1987, Thomas E. Barry documents how the AIDA model actually evolved from the earlier AID model, and then various variants emerged including AIDAS (AIDA + Satisfaction), AIDCA (with the “Conviction” stage added before Action), and similar.
Modern systems also commonly cause variants. For example, AARRR often gets modified by reordering Referral earlier or later in the sequence, or removing it altogether (see the Pirate Metrics Variants for more information). Various authors will claim that their model is somehow better than the original, but bear in mind that this is highly contextual.
In addition to Barry’s article, the Some Insights in the Historical Prospective of Hierarchy of Effects Model: A Short Review article by Rehman and colleagues provides a good overview of historical models and their evolution.
Applicability of Hierarchy of Effects Systems
Hierarchy of Effects systems such as AIDA (Attention, Interest, Desire, Action) and REAN (Reach, Engage, Activate, Nurture) are widely used in designing marketing campaigns. These models help marketers structure communications and interactions with potential customers. For example, in a digital marketing funnel, AIDA can guide content creation, ensuring that advertising captures attention, engages interest, builds desire, and calls for action.
Hierarchy of Effects systems are useful for mapping user journeys in product design. A model like AARRR (Acquisition, Activation, Retention, Referral, Revenue) is particularly effective in the startup world to track and optimize customer engagement. For example, tracking AARRR metrics might help to spot bottlenecks and where users struggle from the moment they download a mobile application (Acquisition) to when they make their first purchase (Revenue).
In general, Hierarchy of Effects models models are useful as an inspiration for developing key performance indicators to track user progress towards product goals. Metrics tied to each step a Hierarchy of Effects system can provide meaningful insights, spot trends, outliers and bottlenecks. For example, in the REAN model, Reach might correspond to the number of impressions or clicks from a specific channel, and if that number suddenly goes up, product managers can modify the product to exploit that opportunity.
Hierarchy of Effects models can (and should) be reconfigured or modified to suit specific product needs. For instance, AARRR, originally created for startups, is often adapted for larger enterprises by expanding the “Retention” stage to include multiple user satisfaction and loyalty metrics and removing the “Referral” stage.
Limits of Hierarchy of Effects Systems
One major limitation of Hierarchy of Effects models is their focus on an idealized, linear flow of customer behavior. In reality, users rarely follow such neat paths. For instance, a customer might jump directly from awareness to purchase, skipping the engagement steps in the product designed for interest and desire phases. Tracking the customer through the funnel directly in each step will create inconsistent data for such exceptions. The AIDA model, for example, struggles to account for impulse buying or repetitive purchasing behaviors. In such cases, the model oversimplifies the complex nature of customer decision-making processes.
The models are highly context-dependent, so they may need to be adjusted for different market segments or industries. For example, a high-tech SaaS product might require a longer and more complex engagement process than a consumer retail product, where the AIDA model may not fully capture the nuances of technical decision-makers in the B2B space.
These models should not be taken too literally, for example assuming that all users move through the same stages at the same pace. For instance, in a subscription-based business model, some users may reach the referral stage early, while others may churn after activation without progressing further. If a metrics system designed on the AARRR model tries to strictly follow users through the funnel, it might not capture these edge cases well, limiting its effectiveness in understanding why certain users deviate from the norm.
Another limitation is that these models often overlook cyclical or repeated behaviors. In reality, users may revisit stages multiple times. For example, in a long-term B2B sales cycle, the process of engagement may be recursive, where clients enter and exit different stages multiple times before making a purchase decision. Hierarchy of Effects systems like REAN, designed to be linear, are less suited to capturing this back-and-forth movement.
While the creation of variants or custom models (such as adding steps to AARRR) allows for broader application, it can also dilute the simplicity and clarity that makes these models popular in the first place.
Learn more about the Hierarchy of Effects
- The Development of the Hierarchy of Effects: An Historical Perspective, Journal of Current Issues and Research in Advertising by Thomas E. Barry (1987)
- A Model for Predictive Measurements of Advertising Effectiveness, Journal of Marketing, Vol. 25, No. 6 by Robert J. Lavidge, Gary A. Steiner (1961)
- Some Insights in the Historical Prospective of Hierarchy of Effects Model: A Short Review, Journal of Information Management and Business Review, Vol. 6 by Fazal ur Rehman, Farwida Javed, Tariq Nawaz, Ishfaq Ahmed, Shabir Hyder (2014)
- Advertising’s sequence of effects on consumer mindset and sales: A comparison across brands and product categories by Albert Valenti, Gokhan Yildirim, Marc Vanhuele, Shuba Srinivasan, Koen Pauwels (2023)
- The trilogy of mind: Cognition, affection, and conation, Journal of the History of the Beavioral Sciences by Ernest R. Hilgard (1980)